En los últimos años se han generalizado programas que intentan predecir qué objetos serán de interés para el usuario, disponiendo de cierta información sobre su perfil. Hasta 2006, estos algoritmos no eran populares. Pero todo eso cambió en el otoño de 2006 cuando Netflix ofreció a los desarrolladores $ 1,000,000 por el mejor algoritmo de predicción. La competición duró 3 años.
Hablemos hoy de nuestra experiencia en la construcción de un sistema de recomendación en la formación de personal.
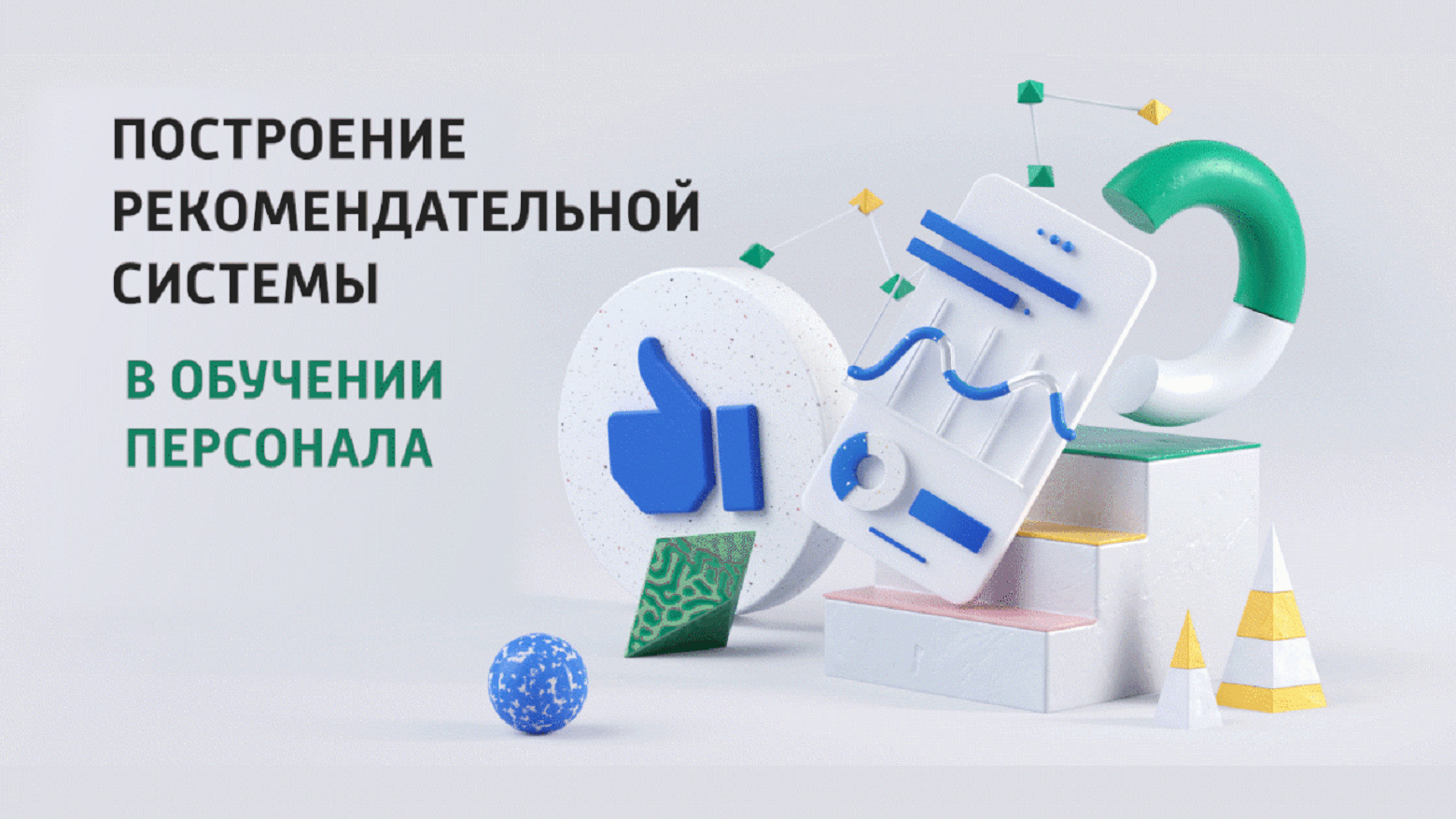
.
?
– IT- . , . . , , .
. . , .
1. Content-based filtering ( )
, . , .
2. Collaborative filtering ( )
, .

3. ,
– . , .
?
. Users. , , Users.
. ( ). , , . …
features ( ) Users.
Users :
/ ( );
/ ;
;
(, Data Analist, Data Engineer, Data Scientist);
( );
( ).
Users .
MVP , . . Users :
(-1, +2);
– ;
– ;
– Data Scientist;
– 5 ( 20 65);
- 5 .
Users – 3 .
– 6 ( 2 User).
– Python.
(DataSet), , , Users.
User 3 Users .
# DataSet
for row in df:
corrMatr = df.corrwith(df[row]) #
corrMatr = pd.DataFrame(corrMatr)
tempMatr = corrMatr #
tempMatr = tempMatr.drop([row], axis=0)
li = list()
li2 = list()
print(row)
k = 0
while k < 6:
if len(tempMatr) == 0: # tempMatr 0, while
break
name = tempMatr.idxmax().item() #
dp = df3[df3['Tab'] == name].set_index('Tab') # ,
# Tab name
if name not in li2 and ((df[name]['pos'] <= df[row]['pos'] + 2 and df[name]['pos'] >= df[row]['pos'])):
#
li2.append(name)
col_dp = dp.columns.tolist() # DataFrame
random.shuffle(col_dp) #
for yy in col_dp: #
if pd.DataFrame(df3[df3['Tab'] == name][yy]).reset_index()[yy][0] == 1 and \
pd.DataFrame(df3[df3['Tab'] == row][yy]).reset_index()[yy][0] == 0 and \
yy not in li and yy in df777[''].tolist():
#
recList.append([row, name, yy,
pd.DataFrame(df4[df4['Tab'] == row]['TB']).reset_index()['TB'][0], \
pd.DataFrame(df4[df4['Tab'] == name]['TB']).reset_index()['TB'][0], \
pd.DataFrame(df4[df4['Tab'] == row]['FIO']).reset_index()['FIO'][0], \
pd.DataFrame(df4[df4['Tab'] == name]['FIO']).reset_index()['FIO'][0]])
k += 1
li.append(yy)
# tempMatr
tempMatr = tempMatr.drop([tempMatr.idxmax().item()], axis=0)
break # for
else: # tempMatr
tempMatr = tempMatr.drop([tempMatr.idxmax().item()], axis=0)
# DataFrame Excel
recomendations = recomendations.append(recList, ignore_index=True)
recomendations.to_excel('.xlsx')
.

. :
(, );
.
.
Este algoritmo de recomendación se implementó en modo piloto (durante un trimestre). El MVP creado ha alcanzado la tasa de conversión objetivo del 25% establecida por la gerencia, lo que nos permite reconocerlo como exitoso y listo para su implementación en la industria.